A Review of Racial, Socioeconomic, and Ableist Bias in the Field and Future of Brain-Computer Interfaces
Rhheaa Mehta, University of Pittsburgh
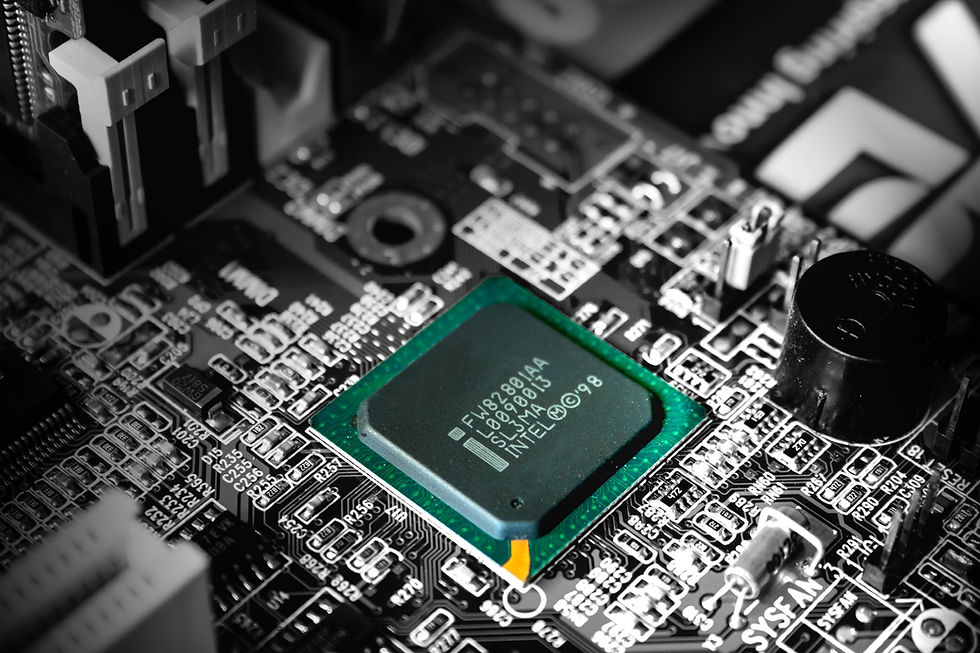
Abstract: Brain-computer interfaces (BCIs) are direct links between brains and computers, aiming to improve the quality of life for people with neurological disorders or traumatic injuries. Despite existing BCIs like cochlear implants or EEGS that revolutionized the field, many biases rooted in the development and application must be diminished for meaningful progress, especially in coordination with AI technology.
Training AI with data that does not consider diverse perspectives can lead to discriminatory outcomes. Racial bias is highly prevalent in AI and deep learning systems, demonstrated in a recent study. As BCIs apply AI to build autonomy, it is critical to mitigate biased responses at every step.
AI inclusion, patents, and market exclusivity can severely limit accessibility through socioeconomic bias. Similar to medications like insulin, lifesaving technologies can have unattainable prices due to modern economic culture. For advanced technology like BCIs, the cost of scalability and algorithmic privacy protections further decrease monetary accessibility. Socioeconomic bias must always be scrutinized in healthcare.
The stigma and ableism inherent to sociocultural perspectives on medical technologies cause many ethical and moral issues not easily resolved. The controversy surrounding technologies like cochlear implants is a clear example of the moral responsibility surrounding BCI technology.
To improve the field, appropriate ethical and social regulations for neurotechnology must be coordinated. Historical issues and current biased results must guide a better foundation to diminish bias and produce meaningful changes in the quality of healthcare and life.
Introduction to BCIs
Brain computer interfaces (BCIs) are neural-system-based interfaces that connect a brain with a computer, both mono- and bidirectionally (Barnova et al., 2023). BCIs work by either extracted endogenous brain signals relating to the user’s mental processes (ex: using brain signals to move an external device) or allowing stimulation of brain nerve tissue in a patterned way (ex: with deep brain stimulation [DBS] for Parkinson’s Disease) (Gao et al., 2021).
The many kinds and categories of BCIs depend on the function that is being differentiated or emphasized. The control category defines the type of stimuli that BCIs react to: active BCIs are controlled by direct and conscious brain activity, reactive BCIs are controlled indirectly by neural stimuli, and passive BCIs are controlled by spontaneous brain activity without any specific or special stimuli (Bergeron et al., 2023). The invasive category defines the machine itself: invasive BCIs have electrodes implanted within the skull, such as electrocorticography; non-invasive BCIs have only external electrodes, such as functional MRIs (Barnova et al., 2023). The decisional category defines the amount of control the user has over the decisions made by the BCI: in-the-loop decisional BCIs allows users to have some control; out-of-loop decisional BCIs gives the user no control over BCI function or activity (Gilbert et al., 2023).
Immediately, the opportunity for bias inherent within the mechanism of implantation and decision-making, affecting risk (ex: surgical, socioeconomical) and autonomy (reflecting decision-making control or lack thereof) become noticeable.
Finally, a brief history of BCI technology allows us to build a greater understanding of the rapidity at which the field is expanding (Gao et al., 2021). In 1924, Hans Berger invented the first electroencephalogram (EEG). In 1973 James Vidal officially coined the term BCI. Med-El created the very first cochlear implant in 1982. DBS was invented and first used for Parkinson’s Disease in 1987. The first human trial of a motor BCI was conducted in 2004 by BrainGate. And in 2023, Willet and Metzger built and successfully trialed a high-performance speech neuroprosthesis (Willet et al., 2023).
The growing fame and popularity of BCI technology, exacerbated by media articles on companies like Elon Musk’s Neuralink and popular culture depictions of sci-fi, is mirrored by similar fame and booming popularity of AI technology. With ChatGPT, AI technology has launched to the forefront of popular culture, but it has held an ever-growing role in other technology for a long time prior, including BCIs.
The Growing Role of AI
Brain signals are non-linear, non-stationary, irregular, and chaotic. A large amount of computing power is necessary to sort through them and ensure that the correct signals are being read by the computer. AI can minimize the computational demand, improve detection accuracy, and improve the transfer rate of brain signals to the computer interface itself (Barnova et al., 2023).
AI technology uses machine learning (ML) and deep learning (DL) techniques to recognize patterns, process raw data brain signals directly, and capture high-level features and latent dependencies (Albahri et al., 2023). Often, EEGs and other non-invasive BCIs use AI to make themselves easier to read and interpret. Additionally, to address the issue of insufficient data in certain datasets, current algorithmic advances have reduced the need for data acquisition and calibration. (Albahri et al., 2023).
AI can minimize issues within BCI equipment during calibration or noise suppression to create clearer images. Mental condition estimation can ensure user/patient readiness and minimize issues stemming from unprepared users. Due to AI’s ability to comprehend large data sets quickly, it can clarify motor imagery and communication. And, of course, it can execute common practical tasks associated with BCIs with relative ease, freeing up machinery and computing power for more impressive tasks (Barnova et al., 2023).
However, AI requires high quality extraction and many network hyperparameters to ensure that it works to the best of its ability (Albahri et al., 2023). BCI technology must be easily portable and usable in everyday scenarios, where high quality data is not always assured. This hindrance limits the efficiency of AI integration. However, burgeoning optimization techniques inspired by nature are increasing AI capabilities to manage large data sets with fewer hyperparameters and lower quality data (Barnova et al., 2023). Additionally, MT-BCI is a growing, multinational effort to collect the largest existing samples of BCI data to create a database that scientists can use to improve AI in BCI technology.
The benefits of AI integration in BCI technology are often diminished by extra parameters that must be worked into new technology to ensure the AI works efficiently. However, the combination of the two can continue to progress the field of BCI further than ignoring AI altogether, as it can significantly benefit data processing and interpretation. If biases are mitigated and acknowledged at every step of integration, AI can only benefit a BCI.
AI Training and Databases
Most AI models required large amounts of data to learn. Without large data sets, AI fails to sufficiently correlate signals and communicate with both the data and the interface. Decision making requires length training, which itself requires even larger data sets. However, with the lack of standardization in current BCI data, there is not enough data upon which to train AI (Barnova et al., 2023). Additionally, algorithms are only tested on data set signals, which do not reflect real-time effectiveness and lead to inauthentic correlations due to overfitting (Tian et al., 2021). Variability in brain anatomy and communication decreases generatability, additionally hampering AI integration and application in BCI technology (Albahri et al., 2023).
Size is not the only aspect of data that needs to be carefully considered. If the training data is non-diverse, limited to specific populations or groups of people, or otherwise homogenous, AI is prone to racial bias in the decisions it makes. Like the human psychological theory of other-race effect, which defines the human tendency to recognize and remember faces within their own race better than those of other races, AI tends to form racially biased decision-making pathways when trained on racially biased data.
Because BCI studies are not standardized, non-uniform, and scattered among many different populations (Barnova et al., 2023), this results in a major bias that must be accounted for. BCI technology is intended to use the neural signals of users to perform tasks or to perform tasks upon the neural signals of users. Racial bias could lead to misinterpretation and misattribution of those neural signals, causing the BCI to execute a task that was not intended. The autonomy of a user is thus brought into question due to the racial bias of the device meant to ameliorate their autonomy in the first place. Additionally, racial bias can cause unintended harm that brings more questions about safety and invasive devices.
Though the solution seems simple (make larger, more diverse data sets!), there are many impediments. Large data sets are just half the equation. Preventing racial bias in training data requires diversity in clinical trials, which is often overlooked. Clinical trials have historically enrolled predominately white test subjects. Even now, despite both 1992 and 2012 US laws, the diversity of clinical trials has not significantly changed (Goering et al., 2021). Additionally, the lack of standardized methods, classifications, and other data collection tools can prevent the data sets from being usable even if the clinical trials are perfectly diverse. And, of course, the complexity and cost of recording neural signals, as well as the invasiveness of the procedure, leads to ethical and socioeconomic dilemmas of informed consent, and who can afford to pay for the procedures (leading again to biased data sets due to systematic and generational socioeconomic status) (Barnova et al., 2023).
Potential solutions also lie in changing the process of AI training altogether. Rather than balancing data sets with data collection, back-propagation algorithms could be revised. Utilizing multi-head deep convolution neural networks (DCNNs) with one head for target classification, one for removing biased learned attributes, and other heads for other aspects can retroactively minimize racial bias (Barnova et al., 2023). Additionally, scientists can work towards actively fighting biased data collection and proactively prevent racial bias (Goering et al., 2021).
Racial bias pervades not only every field, but every aspect of every field. AI output will not be biased if the training input is not biased, which will not be biased if the data is not biased, which can only happen if researchers are actively fighting racial bias from the very beginning. Of course, some issues (such as socioeconomic problems that can create racially biased datasets) are more systematic, but other factors that can be controlled must be, and even those that seem out of our hands must at least be attempted to be controlled.
Racial bias pervades every aspect of the process of AI training. The AI output can only produce biased decisions if the training input was biased. The training input can only be biased if the data collection was biased. And thus, researchers are reminded that it is not enough to passively or retroactively diversify and remove racial bias, but it must be actively worked at from the beginning of every project.
It is not enough to merely consider variables like systematic socioeconomic issues that may preclude involvement of certain racial groups, or what groups would make a representative sample within the experiment. These variables must be actively worked into the methods and procedure of trials and accounted for at every step within an experiment before it even makes it to the public. Racial bias can be easily ignored, but it should not continually slip by the wayside, especially in cases that will later effect autonomy and care of impacted individuals with BCIs.
Security and Accessibility
BCI technology is hampered by the robust security and privacy measures that are required to ensure the safety of patient data. Input neural signals contain private and sensitive medical and personal information that must be protected under HIPPA and is thus forced into the same stringent security measures (Zhang et al., 2019). Most BCI also have unrestricted access to users’ brainwaves, with the potential to infer private information from those neural signals. Identification, inference, model inversion, and even extraction attacks are all highly anticipated upon BCI technology itself (Xia et al., 2022).
Security measures to prevent such attacks are critical to implant before BCI technology can become widely used. Several solutions have already been found, such as source free transfer learning, anonymization, data sanitization, and cryptography-based approaches can prevent attacks at communication ad interface levels (Xia et al., 2022). However, these approaches are all expensive. They demand high computational complexity and power, trusted hardware, complex machine learning, third party data centers, and time. To offset the cost of security, the price of the technology itself would be increased. The raised prices immediately build socioeconomic bias, as putting an expensive price on essential technology creates barriers that are impossible to cross for users in lower socioeconomic brackets.
Already, direct-to-consumer neurostimulation devices are used in much higher income brackets relative to the general population. For example, access to DBS in Canada for people with advanced Parkinson’s Disease varies widely from province to province, depending on income and socioeconomic class of the patients (Bergeron et al., 2023).
Artificial price gouging in other medical technologies already negative impact access to said technologies that can be used as an example to avoid in the BCI field. For example, policies intended to contain costs of medicines like insulin still adversely impact appropriate medical access. While generic medications can improve market competition as they tend to be less expensive, BCI technology inherently cannot have generic brands of medicine due to the nature of the computer technology itself and the high barriers into the field of development and engineering of BCI technology. Additionally, the limited flexibility of the federal government decreases its negotiation efforts and increases lack of transparency in said negotiations, which often do not result in meaningful change (Herman & Kuo, 2021).
The perpetuation of these issues in a wide variety of fields demonstrates the higher chances of these same biases populating of BCI technology. However, there are some solutions at a policy level that may prevent artificial price gouging if implemented effectively. Increasing competitive pressure and revising loopholes in the 180-day exclusivity period of patents could increase competition and drive prices down. Additionally, emphasizing greater transparency and flexibility on the part of the federal government in negotiating healthcare policy could decrease price gouging (Herman & Kuo, 2021).
In medical technology, one must always consider the role of socioeconomics in the accessibility and availability of technology. Disabled people who could benefit from BCI technology often cannot afford it, especially as many must keep their income low to access other benefits from the government already. The duty of the healthcare field is to ensure that those most vulnerable have access to what they need, and actively working against creating inaccessible technologies or price gouging vital technologies is an important part of creating a field that can progress and make meaningful impact on people’s lives.
Agency, Identity, and Culture
Technology that works primarily with disabilities often walks a fine line between aid and ableism. This is reflected in quotes by BCI developers, who justify their works as a response on “personal, social, and economic burdens of [user] disabilities” (Sample et al., 2023). The emphasis on burden rings many alarm bells, all of them chiming with the same tone of technoableism.
Coined by Dr. Ashley Shew, technoableism encompasses the concept of simultaneous discussion of empowering disabled people through new technologies while reinforcing ableist tropes about what a “normal” brain functions like and who is worthy of participating in society (Shew, 2023). The obligations of the ‘sick role’, devised by Talcott Parsons in the 1950s, is for the sick individual to see sickness as undesirable, and do everything within their power to get better and come back to work in a functional society. The chronically disabled are thus seem as avoiding the obligations of this socially constructed sick role and thus must be ‘fixed’ to ensure society can continue to run. This is especially seen in modern American society, where the medical model of disability is prevalent, and the conceptual understanding of traits as disabilities is intrinsically related to the economic value of one’s labor on the market (Shew, 2023).
A clear example of technoableism in the field of BCI was the introduction of cochlear implants (CIs) to the market. They were pushed onto parents of deaf or hard of hearing children, explained as technology that would “fix” their children and ensure their smooth integration back into hearing society, which they lost when they failed their hearing test. Worried parents, scared of a future of hardship and inability for their children, accepted trustingly what authoritative doctors told them. These instructions often included and, in fact, emphasized neglecting to teach ASL or Deaf culture, which would minimize the effects of the CIs (Bergeron et al., 2023).
The culture around CI technology may have changed recently, through decades of Deaf activism and education, and is now often encouraged alongside ASL and Deaf culture. However, there are many other examples, such as the advance of CRISPR technology for selective abortion of fetuses that might develop autism (Shew et al., 2023) and exoskeletons to “rescue” paralyzed people “bound” to their wheelchair (Barnova et al., 2023), where the sick role theory comes yet again into play.
The attitude of those doctors and professionals who push new technologies onto disabled people (or their parents by proxy) enforce the idea of disabled autonomy only as long as it functions within the goal of fixing or removing their disability so that they can go back to being productive members of society. The constant discrimination against those disabled people who chose to go against or completely disregard the medical model of disability and the sick role is treated as inexplicable and antisocial.
Experiments show that those who do chose to refuse technology that could ‘fix’ them, such as a BCI, even hypothetically, are treated with more blame, anger, and coercion than those who accept the BCI. Despite any external validity of reasons, such as socioeconomic or health risks, participants treat the individual with blame and anger, turning the cause of the problem internally onto the victim rather than the situation (Sample et al., 2023).
This attribution theory of blame shows the inherent technoableist perspective of technology as the end-all-be-all ‘fix-it’ of disabilities. It disregards external situational factors and attributes the problem of the disability onto the individual themselves, for not choosing to fix themselves how other think they should. When able society defines autonomy in this narrow way (autonomy only to fix one’s disability), it prevents other ethical concerns regarding autonomy from being widely discussed, especially if those concerns rely within the pushed technologies themselves.
The black box problem of AI is the issue that no one quite understands how AI makes the decisions that AI does. While the input and output are well understood and utilized, the decision-making process itself is a complete mystery, which is a concern when that decision-making process is interacting with a person’s neural signals and body to interact with their environment. Is it true autonomy or is the AI the one making the decisions for the individual? How could one pinpoint who is making the decisions, or if the decisions being made are adapted or twisted due to irrelevant or biased features of the AI (Gombolay et al., 2023)?
Inherent to the black box problem is the threat posed to human identity, agency, and privacy. BCI users adapt to the neuroprosthesis as any other prosthesis to make it an extension of them. They become unsure of “what’s me and what’s the depression and what’s the stimulator” as a patient with a BCI for treatment resistant depression phrased it. As BCIs are integrated into the user’s identity and being, it can be hard to separate out the agency of the individual from the decision-making capabilities of the AI, which is worrisome when coupled with the lack of knowledge about those processes altogether (Bergeron et al., 2023).
An extreme example of the integration and ethical issues that it can cause come from a trial for epilepsy treatment BCIs. Patient R was implanted with a BCI and gained great de novo agential capacity inseparable from her device. Her of-the-loop abilities increased self-discovery, self-definition, and self-direction, building existential dependency on her BCI. But as the company went bankrupt, the resultant forced explanation caused radical psychological discontinuation, disruption of agency, grief, and lost feelings of symbiotic agency (Gilbert et al., 2023).
Suppose the implanted BCI, which in and of itself created such existential angst, also had AI integrated capabilities. How much worse could the damage have been, and how much could be ethically allowed for the purpose of ‘fixing’ the patient before the side effect became too extreme to countenance?
The current social and medical attitudes of the obligations of the sick role and the medical model of disability create a hegemony reliant on pushing technology onto populations that may not want it. Despite the many external factors that affect someone’s decision to accept or decline BCI technology, the blame is placed on the individual, even despite the risk of existential angst at the potential of explantation. The ethical bias towards this medical model must be examined and actively considered in every situation during the production and prescription of BCI technology to an individual.
Discussion
The racial, socioeconomic, and ableist biases within BCI technology leads to ethical concerns with the production, accessibility, and prescription of said devices. As the field grows, integrating with AI and other computational techniques, it is critical to focus on the effects of such technology on healthcare and the people they are trying to benefit, rather than society as a whole. Ensuring unbiased and standardized datasets, privacy and security measures that do not lead to price gouging, and societal perceptions that do not reflect ableist views or major ethical concerns are integral to creating BCI technology that will actually benefit and support its users.
The technological advances in medicine often leave behind those who would benefit from this new technology. Modern American healthcare creates holes that people of low socioeconomic status, racial and ethnic minorities, and anyone that does not fit into a specific mold of individual fall through. The inequity is self-perpetuating and generational, as each new generation of doctor, nurse, and healthcare professional learns from the generation before them and from societal expectations to lean onto the medical model as the best and most viable model for all.
Proceeding further must come with the idea of “do no harm” at the forefront and the reminder that good intentions do not erase actual effects. Rather than passively discussing or retroactively changing problems that arise, active participation in every aspect of BCI technology, from the datasets that train them to the actual application and prescription of them, must consider a holistic understanding of the individual that acknowledges and thus fights against racial, socioeconomic, and other ethical biases.
The idea improvement would be appropriate ethical and social regulations for neurotechnology, combining the fields of medicine, law, and social justice. Learning from historical and current issues to create a better foundation for a future with diminished bias and improved quality is vital. However, just as often as the law can, at the very least, provide those with disabilities a jumping point to advocate for themselves, it can also create an endless black hole of bureaucracy that traps individuals without getting effective help or meaningful change. Thus, progress in the world of healthcare comes in two parts: through both the establishment of effective and meaningful legislature at every level of government and the active work of those researchers and healthcare professionals who develop and give the BCI technologies to individuals to use.
References
Albahri, A. S., Al-qaysi, Z. T., Alzubaidi, L., Alnoor, A., Albahri, O. S., Alamoodi, A. H., & Bakar, A. A. (2023). A Systematic Review of Using Deep Learning Technology in the Steady-State Visually Evoked Potential-Based Brain-Computer Interface Applications: Current Trends and Future Trust Methodology. International Journal of Telemedicine and Applications, 2023, 1–24. https://doi.org/10.1155/2023/7741735
Barnova, K., Mikolasova, M., Kahankova, R. V., Jaros, R., Kawala-Sterniuk, A., Snasel, V., Mirjalili, S., Pelc, M., & Martinek, R. (2023). Implementation of artificial intelligence and machine learning-based methods in brain–computer interaction. Computers in Biology and Medicine, 163, 107135. https://doi.org/10.1016/j.compbiomed.2023.107135
Bergeron, D., Iorio-Morin, C., Bonizzato, M., Lajoie, G., Orr Gaucher, N., Racine, É., & Weil, A. G. (2023). Use of Invasive Brain-Computer Interfaces in Pediatric Neurosurgery: Technical and Ethical Considerations. Journal of Child Neurology, 38(3–4), 223–238. https://doi.org/10.1177/08830738231167736
Brain–computer interface. (2024). In Wikipedia. https://en.wikipedia.org/w/index.php?title=Brain%E2%80%93computer_interface&oldid=1216770525
Gao, X., Wang, Y., Chen, X., & Gao, S. (2021). Interface, interaction, and intelligence in generalized brain–computer interfaces. Trends in Cognitive Sciences, 25(8), 671–684. https://doi.org/10.1016/j.tics.2021.04.003
Gilbert, F., Ienca, M., & Cook, M. (2023). How I became myself after merging with a computer: Does human-machine symbiosis raise human rights issues? Brain Stimulation, 16(3), 783–789. https://doi.org/10.1016/j.brs.2023.04.016
Goering, S., Klein, E., Specker Sullivan, L., Wexler, A., Agüera y Arcas, B., Bi, G., Carmena, J. M., Fins, J. J., Friesen, P., Gallant, J., Huggins, J. E., Kellmeyer, P., Marblestone, A., Mitchell, C., Parens, E., Pham, M., Rubel, A., Sadato, N., Teicher, M., … Yuste, R. (2021). Recommendations for Responsible Development and Application of Neurotechnologies. Neuroethics, 14(3), 365–386. https://doi.org/10.1007/s12152-021-09468-6
Gombolay, G. Y., Gopalan, N., Bernasconi, A., Nabbout, R., Megerian, J. T., Siegel, B., Hallman-Cooper, J., Bhalla, S., & Gombolay, M. C. (2023). Review of Machine Learning and Artificial Intelligence (ML/AI) for the Pediatric Neurologist. Pediatric Neurology, 141, 42–51. https://doi.org/10.1016/j.pediatrneurol.2023.01.004
Herman, W. H., & Kuo, S. (2021). 100 years of insulin: Why is insulin so expensive and what can be done to control its cost? Endocrinology and Metabolism Clinics of North America, 50(3 Suppl), e21–e34. https://doi.org/10.1016/j.ecl.2021.09.001
Sample, M., Sattler, S., Boehlen, W., & Racine, E. (2023). Brain-computer interfaces, disability, and the stigma of refusal: A factorial vignette study. Public Understanding of Science, 32(4), 522–542. https://doi.org/10.1177/09636625221141663
Schermer, M. (2009). The Mind and the Machine. On the Conceptual and Moral Implications of Brain-Machine Interaction. NanoEthics, 3(3), 217–230. https://doi.org/10.1007/s11569-009-0076-9
Shew, A. (2020). Ableism, Technoableism, and Future AI. IEEE Technology and Society Magazine, 39(1), 40–85. https://doi.org/10.1109/MTS.2020.2967492
Simon, C., Bolton, D. A. E., Kennedy, N. C., Soekadar, S. R., & Ruddy, K. L. (2021). Challenges and Opportunities for the Future of Brain-Computer Interface in Neurorehabilitation. Frontiers in Neuroscience, 15, 699428. https://doi.org/10.3389/fnins.2021.699428
Tian, J., Xie, H., Hu, S., & Liu, J. (2020). Multidimensional face representation in deep convolutional neural network reveals the mechanism underlying AI racism. https://doi.org/10.1101/2020.10.20.347898
Willett, F. R., Kunz, E. M., Fan, C., Avansino, D. T., Wilson, G. H., Choi, E. Y., Kamdar, F., Glasser, M. F., Hochberg, L. R., Druckmann, S., Shenoy, K. V., & Henderson, J. M. (2023). A high-performance speech neuroprosthesis. Nature, 620(7976), Article 7976. https://doi.org/10.1038/s41586-023-06377-x
Xia, K., Duch, W., Sun, Y., Xu, K., Fang, W., Luo, H., Zhang, Y., Sang, D., Xu, X., Wang, F.-Y., & Wu, D. (2023). Privacy-Preserving Brain–Computer Interfaces: A Systematic Review. IEEE Transactions on Computational Social Systems, 10(5), 2312–2324. https://doi.org/10.1109/TCSS.2022.3184818
Zhang, X., Ma, Z., Zheng, H., Li, T., Chen, K., Wang, X., Liu, C., Xu, L., Wu, X., Lin, D., & Lin, H. (2020). The combination of brain-computer interfaces and artificial intelligence: Applications and challenges. Annals of Translational Medicine, 8(11), 712. https://doi.org/10.21037/atm.2019.11.109
Comments